Introduction
Many diseases that threaten human life come from bacteria, parasites, and viruses (1-3). Furthermore, these bacteria, parasites, and viruses can be transmitted through animals (4-6). One of them is malaria. Malaria is a disease that threatens human life. It is caused by a parasite transmitted to humans by biting an infected female Anopheles mosquito (7). The transmission of this disease can be prevented and cured. There are five species of parasites that cause malaria in humans. Two parasitic species, namely Plasmodium falciparum and Plasmodium vivax, are the most significant threats (8-10).
The risk of malaria will affect around half of the world's population by 2020. Sub-Saharan Africa has the greatest number of cases and fatalities. In the World Health Organization (WHO) regions of Southeast Asia, the Eastern Mediterranean, the Western Pacific, and the Americas, several cases and fatalities were reported. In 2020, there will likely be 241 million instances of malaria and 627,000 fatalities, according to estimates. a WHO Globally, the burden of malaria is disproportionately high throughout the African continent (7, 11). The percentage of malaria cases and fatalities in the region by 2020 will reach 95% and 96%, respectively. The WHO Africa Region predicts that by 2020, children under the age of five will account for about 80% of all malaria deaths. The top cause of death in the world is malaria. Malaria is a disease that affects the tropical and subtropical regions of the earth between 60 degrees north and 40 degrees south. Over 2.3 billion individuals, or 41% of the world's population, are susceptible to malaria. Between 300 million and 500 million people contract malaria annually, which results in 1.5 million to 2.7 million fatalities (12, 13).
Malaria has been successfully eradicated worldwide. In this time, malaria prevention and control initiatives have helped save at least 1.1 million lives (14). In high-epidemic areas, it is still challenging for people to receive effective malaria prevention, diagnosis, and treatment. Currently, funding is available for initiatives to lower malaria incidence and prevalence globally (3, 15). To create an efficient and effective malaria control program, it is essential to comprehend the efficacy and effectiveness of control methods. Malaria mathematical modeling enables precise forecasting of the program's utilization of control methods (16).
In the last ten years, articles related to mathematical models of malaria transmission involving human and mosquito populations have been discussed in (14, 17-20). The mathematical model of malaria transmission continues to be developed, involving the optimal control strategy discussed in (21-23). Control of malaria transmission can also be done by analyzing the effect of each parameter in the model, as discussed in (8, 24, 25). The mathematical model of malaria transmission discussed in this article adopts the model from Xing et al. (26-29). The discussion in (30, 31) studies focuses solely on the model's backward bifurcation and the equilibrium point's global stability; no attention is paid to the sensitivity analysis of any individual model parameter. The sensitivity of each parameter in the model influences the precision of future predictions of malaria transmission. Finding the malaria transmission model's most important parameters requires conducting a sensitivity study. When developing ways to prevent malaria transmission, these factors might be utilized as a guide. Consequently, the objective of this discussion is to assess the sensitivity index of the mathematical model of malaria transmission from (26).
Materials and Methods
Mathematical modeling for the problem of malaria transmission is a theoretical study. The discussion in this article is focused on analyzing the sensitivity of the malaria transmission model. The method used in the discussion of this article is a theoretical study method with references from several reputable international articles. The flow of discussion in this article begins with constructing a malaria transmission model, determining the equilibrium point, and determining the basic reproduction number and the sensitivity index of each parameter in the model. Finally, a numerical simulation is given to qualitatively review the influence of the most dominant parameter changes on the human population infected with malaria.
Results
The mathematical model of malaria transmission in this discussion refers to the article by (30). The population studied for the incidence of malaria transmission was divided into three human compartments and two adult female mosquito compartments. A Susceptible Infected Recovered (SIR) deterministic model is formulated for the human compartment. The number of humans who are healthy and susceptible to malaria at the time t is denoted by Sh(t)
, and the number of humans infected with malaria at the time t
is denoted by Ih(t)
, and the number of humans who are cured at the time t is denoted by Rh(t)
. If the number of the individuals population at time t is denoted by Nh(t)
, then Sht+Iht+Rht=Nh(t)
. The SI (Susceptible-Infected) deterministic model is formulated for the female mosquito compartment (32). The proportion of vulnerable adult female mosquitoes and those that can be infected with plasmodium parasites at time t is denoted by Sv(t)
, and the number of adult female mosquitoes infected with plasmodium parasites at time t is denoted by Iv(t)
. If the population of adult female mosquitoes at the time t is denoted by Nv(t)
, then Svt+Ivt=Nvt.
The infection period of the adult female mosquito in this model is assumed to last until her death because her life cycle is relatively short. Adult female mosquitoes compete for food and a place to lay eggs because the resources associated with mosquito reproduction are limited. It is expected that the mosquito birth rate is higher than the death rate. Immunity to malaria does not occur permanently in some people. Figure 1 shows a flow chart for formulating a mathematical model of malaria transmission. The mathematical model of malaria transmission is formulated in a system of nonlinear ordinary differential equations as follows (26).
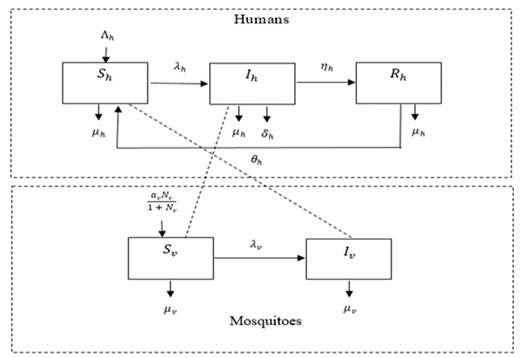
Figure 1. Schematic diagram of SIR model in human population and SI model in mosquito population
dShdt=Λh+θhRh-λhSh-μhSh
dIhdt=λhSh-(μh+δh+ηh)Ih
dRhdt=ηhIh-(θh+μh)Rh
dSvdt=avNv1+Nv-(λv+μv)Sv dIvdt=λvSv-μvIv (1)
with Sht+Iht+Rht=Nht, Svt+Ivt=Nvt,
λv=rβhIhNh
λh=rβvIvNh
The total population of adult female mosquitoes fulfills the following equation
dNvdt=avNv1+Nv-μvNv
Thus, System 1 is equivalent to the following equation
dShdt=Λh+θhRh-λhSh-μhSh
dIhdt=λhSh-(μh+δh+ηh)Ih
dRhdt=ηhIh-(θh+μh)Rh
dIvdt=rβhIhNh(Nv-Iv)-μvIv
dNvdt=avNv1+Nv-μvNv (2)
All parameters in System 2 are positive and av>μv
. The description of all parameters of System (2) is given in Table 1.
The solution of System (2), which does not vary over time, is the equilibrium point of System (2) (constant solution). Suppose P=Sh*, Ih*, Rh*, Iv*, Nv*
is the equilibrium point of System 2, then:
Λh+θhRh-λhSh-μhSh =0 λhSh-(μh+δh+ηh)Ih
=0 ηhIh-(θh+μh)Rh
=0 rβhIhNh(Nv-Iv)-μvIv
=0 avNv1+Nv-μvNv=0 (3)
The equilibrium point has a biological meaning that depends on I*
. If humans and adult female mosquitoes are not infected with malaria in the population Ih*=Iv*=0
, then this state is said to be disease free. On the other hand, if in the population there are humans and adult female mosquitoes infected with malaria Ih*>0, Iv*>0
, then this condition is called endemic. In System 3, for the case of Ih*=Iv*=0
there is one disease-free equilibrium point, namely E0Λhμh, 0, 0, 0, r0μv
.
The basic reproduction number is a threshold to determine whether malaria endemic will occur in the population. The following will determine the basic reproduction number using the next-generation matrix method (30).
Model (2) has two infected states i.e. Ih
and Iv
So we have the following subsystem,
dIhdt=rβvIvNhSh-σ1hIh
dIvdt=rβhIhNh(Nv-Iv)-μvIv
With σ1h=μh+δh+ηh
.
If for example XT=Ih*,Iv*T
where T
is a transpose, then the subsystem can be written as
X=F-VXT
with
F=rβvIvNhSh rβhIhNvNh and V=σ1hIh rβhIhIvNh+μvIv
So that the Jacobian matrix F
and V
di E0
is obtained as follows
F=0 rβv rβhr0μhΛhμv 0 and V=σ1h 0 0 μv
Thus, the basic reproduction number of System 2 based on the following generation matrix method (30) is
R0=ρFV-1=r2βhβvr0μhΛhμv2σ1h
If Ih*>0
dan Iv*>0
, then System 3 obtains the endemic equilibrium point E1Sh*, Ih*, Rh*, Iv*, Nv*
with
Sh*=σ1hσ2hΛhσ1hσ2hλh*+μh-θhηhλh*, Ih*= Sh*σ1hλh* , Rh*= ηhSh*σ2hσ1hλh*
Iv*=λv*r0μvλv*+μv, Nv*=r0μv
λv*=rβhIh*Nh* , λh*=rβvIv*Nh* , σ1h=μh+δh+ηh, σ2h=μh+θh
λh*
is the positive root of the equation
K1λh*2+K2λh*+K3=0 (4)
with
K1=Λhμvσ2h+ηhrβhσ2h+σ2h+ηhμv
K2=MμhLμvσ1hσ2h-θhηh-R02
with
M=Λhμv2σ1hσ2hσ1hσ2h-θhηhμh
L=rβhσ2h+2μvσ2h+2μvηh
K3=Λhμv2σ1h2σ2h21-R02, ∆=K22-4K1K3
The value of K3
in equation (4) depends on R0
so that to determine the endemic equilibrium point, it can be reviewed based on the following two cases:
- If R0<1
, then K3>0
consequently
- If K2>0
, then there is no endemic equilibrium point,
- If K2=0
, then there is no endemic equilibrium point,
- If K2<0
, then for ∆<0,
there is no endemic equilibrium point; for ∆=0, there is one endemic equilibrium point; for ∆>0
, there are two endemic equilibrium points.
If R0>1,
then K3<0
consequently, in System (2), there is one endemic equilibrium point.
Discussion
Table 1 shows all the basic parameter values used in System 2. The parameter value consists of two cases, namely: low transmission R0<1 and high transmission R0>1 . The parameter values are taken from (18). The basic reproduction number for low transmission based on the parameter values in Table 1 is R0=0,75 . The disease-free equilibrium point 2500, 0, 0, 0, 39 was locally asymptotically stable for this case with initial values 2000, 180, 0, 200, 150 . While the basic reproduction number for cases of high transmission based on the parameter values in Table 1 is R0=1,28 . The endemic equilibrium point (71, 16, 18, 5, 11) was locally asymptotically stable for this case with the initial values (300, 10, 10, 200, 150) . Sensitivity analysis provides information on how important each parameter is to the spread of tuberculosis. The knowledge is useful for complex nonlinear model reduction and data assimilation in addition to experimental design. The purpose of this analysis is often to assess the model's robustness or robustness to changes in parameter values. Because errors frequently arise during data collection and in estimated parameter values, this is done.
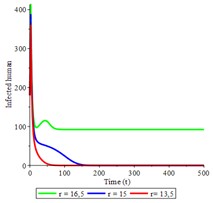
Figure 2. Dynamical Population of infected human for the different values of an individual mosquito's average number of bites on all human hosts parameter
The parameters that have a significant impact on the baseline reproduction rate and should be the focus of the intervention approach are also identified through this research. A sensitivity index is typically used to measure the proportional change in a variable when a parameter changes. The ratio of the relative change in the variable to the relative change in the parameter is known as the sensitivity index of a variable with respect to that parameter. The partial derivative can be used to determine the sensitivity index when the variable is a differential function with respect to a parameter. The sensitivity index is described in the sentences that follow. Definition. The sensitivity index of a variable W that depends on the differentiability of a parameter p is defined as
ΥpW=∂W∂p×pW
This definition means that the higher the sensitivity index, the higher the impact on the measured variable and vice versa. Sensitive parameters must be calculated with caution since even tiny changes will result in significant quantitative changes. In the following, the calculation of the sensitivity index of the basic reproduction number is applied to the parameter βh .
ΥβhR0=∂R0∂βh×βhR0=12
The sensitivity index formulation of the other parameters can be determined analogously as above. The index of sensitivity of the reproduction number to different parameters in System (1) is presented in Table 2. The parameters with a high to low impact on the basic reproduction number are displayed in Table 2 by the sensitivity index. In general, two parameters have the most impact on the basic reproduction number. These parameters include an individual mosquito's average number of bites on all human hosts (r) and the number of mosquitoes that die naturally μv .
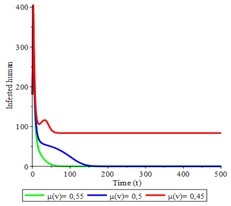
Figure 3. Dynamical Population of infected human Ih for the different values of The number of mosquitoes that die naturally parameter μv when R0<1
Parameters with a positive value of the sensitivity index showed a positive significance in increasing the basic reproduction number. If the value of the r parameter in Table 1 increases while the other parameters remain constant, the result is an increase in the basic reproduction number. Parameters with a negative sensitivity index showed a negative sign in the rise in the basic reproduction number. If the value of the parameter μv in Table 1 decreases while the other parameters remain.
Table 1. The basic values of the parameters used in the malaria transmission model (2)
Parameter |
Description |
Baseline Low |
Baseline High |
βh |
The likelihood of transmission increases with each bite of a vulnerable mosquito. |
0.2 |
0.2 |
βv |
The likelihood of transmission per bite to a vulnerable individual |
0.24 |
0.3 |
r |
An individual mosquito's average number of bites on all human hosts |
15 |
21 |
Λh |
The number of people hired on average |
50 |
21 |
μh |
The number of humans who die naturally |
0.02 |
0.12 |
δh |
The proportion of people who die from disease |
0.5 |
0.5 |
ηh |
Humans' recuperation rates |
0.07 |
0.7 |
av |
The highest value of the viable mosquito egg recruitment rate |
20 |
10 |
μv |
The number of mosquitoes who die naturally |
0.5 |
0.8 |
θh |
The rate at which humans lose their immune |
0.5 |
0.5 |
The result is that the basic reproduction number increases. Table 2 shows an individual mosquito's average number of bites on all human hosts (r) and the number of mosquitoes that die naturally μv are the parameters that contributes the most to the spread of malaria.
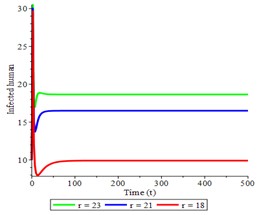
Figure 4. Dynamical Population of infected human Ih for the different values of an individual mosquito's average number of bites on all human hosts parameter (r) with R0>1
This is because the sensitivity index of the parameter r for low and high transmission is greater than the sensitivity index of other parameters. Likewise, the sensitivity index parameter μv for low transmission and high transmission is the lowest value compared to the sensitivity index of other parameters. If the value of the r parameter increases by 10%, it will increase the basic reproduction number by 10.6%. Meanwhile, if the value of the parameter μv decreases by 10%, it will increase the basic reproduction number by 22.6%. In the following, a numerical simulation of System 1 is given to show the effect of variations in the parameters r and μv on the number of infected individuals. If the value of the r parameter for the low transmission case in Table 1 of 15 increases by 10% to 16.5, then the number of infected humans in this situation will increase. Meanwhile, if the value of the r parameter decreases by 10% to around 14.5, then the number of infected humans will decrease.
Table 2. Sensitivity index of basic reproduction number for each parameter in System (1)
Parameter |
Sensitivity Index |
Low transmission |
High transmission |
βh |
0.5 |
0.5 |
βv |
0.5 |
0.5 |
r |
1 |
0.99 |
Λh |
-0.5 |
-0.5 |
μh |
0.24 |
0.45 |
δh |
-0.16 |
-0.19 |
ηh |
-0.08 |
-0.26 |
av |
0.52 |
0.54 |
μv |
-1.02 |
-1.04 |
θh |
0 |
0 |
The numerical simulation is shown in Figure 2. If the parameter value μv for the low transmission case in Table 1 is 0.5, it increases by 10% to 0.55, and the number of infected humans will decrease. Meanwhile, if the parameter value μv decreases by 10% to 0,45 , then the number of infected humans will increase. The numerical simulation is shown in Figure 3. If the value of the parameter r for high transmission cases in Table 1 is 21, it increases by 10% to 23; then, in this situation, the number of infected humans will increase. Meanwhile, if the value of the r decreases by 10% to around 18, then the number of infected humans will decrease. The numerical simulation is shown in Figure 4. If the parameter value μv for high transmission cases in Table 1 is 0.8, up 10% to 0.88, then the number of infected humans will decrease. Meanwhile, if the value of the parameter μv decreases by 10% to 0,72 , then the number of infected humans will increase. The numerical simulation is shown in Figure 5.
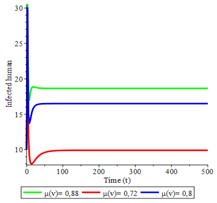
Figure 5. Dynamical Population of infected human Ih for the different values of The number of mosquitoes that die naturally parameter μv when R0>1 .
Conclusion
The sensitivity index shows that the parameter of an individual mosquito's average number of bites on all human hosts (r) is the most dominant in increasing the spread of malaria in humans. On the other hand, the number of mosquitoes that naturally μ v parameter is the most important parameter in reducing the spread of malaria in humans. This information allowed researchers to identify the model's robustness in predicting the number of people infected with malaria. This analysis provides essential information for decision-makers to solve the problem of the spread of malaria.
Acknowledgment
None.
Conflicts of Interest
The authors declared no competing interests.
Author Contributions
This article was written by Saiful Marom, Joko Harianto and Etty Kurniawati. Saiful Marom and Joko Harianto contributed to mathematical modeling, and Etty Kurniawati contributed to malaria transmission. All authors have read and approved the final published version of the article.
Funding
None.